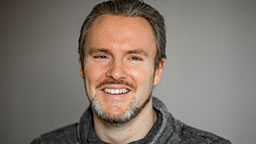
Artificial Intelligence Literacy and Adjacent Digital Literacies for the Digitalised and Datafied Language Industry
A summary of the talk by Professor Ralph Krüger - Institute of Translation and Multilingual Communication at TH Köln - University of Applied Sciences, Cologne, Germany at the International University Network in Technical Communication (IUNTC) on October 31, 2024
Prelude
“Just when we thought that the neural machine translation systems dating from 2016 were the main instance of automation we had to grapple with, the application of large language models (LLMs) to automated text generation […] from late 2022 has brought automated language processes to a new level, not just in translating but also in revising and adapting texts to user-specified receptor profiles. The challenge now is to predict the consequences for long-term translator employment and thereby to adapt our training to a new professional environment.” (Ayvazyan/Torres-Simón/Pym 2024:122)
“There is little doubt that AI reconfigures the distribution of intelligence, labour and power between humans and machines, and thus new kinds of capabilities are needed […].” (Markauskaite et al. 2022:2)
In this talk, Prof. Krüger began with a discussion of the impact of AI in the language industry, outlined AI literacies, and then described the individualdimensions of the AI Literacy Framework for Translation, Interpreting and Specialized Communication.
Prof. Dr. Ralph Krüger, University of Applied Sciences, Cologne - Germany
Ralph Krüger is Professor of Language and Translation Technology at the Institute of Translation and Multilingual Communication at TH Köln – University of Applied Sciences, Cologne, Germany. He received his PhD in translation studies from the University of Salford, UK, in 2014 and completed his habilitation at Johannes Gutenberg University Mainz, Germany, in 2024. His current research focuses on the performance of neural machine translation (NMT) and large language models (LLMs) in the specialised translation process and on didactic strategies and resources for teaching the technical basics of NMT/LLMs to students of translation and specialised communication programmes. ORCID: orcid.org/0000-0002-1009-3365
November 2024 - written by Yvonne Cleary & Daniela Straub
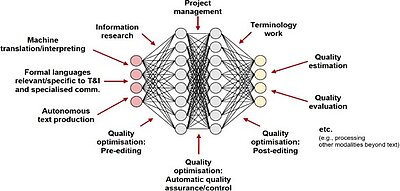
Read full article
Introduction
At one point, at least in the field of translation, it felt like we were at the cutting edge: Neural machine translation was well researched, findings were available, syllabuses and courses had been worked out. But the emergence of ChatGPT means we now have to deal with many new developments. While ChatGPT is not necessarily better at translation than, for example, DeepL, it allows for fine-tuning and customization of output to a much greater degree than ‘traditional’ neural machine translation. Language models open up a wide range of tasks beyond machine translation that can be (partially) automated in language industry workflows.
Recently, we have entered the era of multimodal LLMs. These models, such as GPT-4o, seamlessly integrate different modalities such as text, voice, images and video. Thus, we have moved from purely text-based models to modern multimodal models, which are summarized under "general-purpose AI". The European Parliament Research Service defines general purpose AI as "[M]achines designed to perform a wide range of intelligent tasks, think abstractly and adapt to new situations” (European Parliamentary Research Service 2023:1).
The extended capabilities of these models go far beyond machine translation. As figure 1 below shows, they can be used, among other things, to support project management, terminology work, automatic terminology extraction, quality assessment and control as well as automatic post-editing.
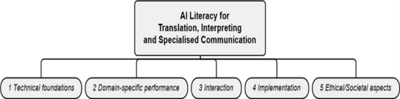
Recording AI skills
Since the invention of the computer, numerous digital skills have emerged which are summarized under terms such as computer literacy, information literacy, media literacy, and data literacy. The collective term for these is digital literacy, which also includes machine translation skills. AI literacy is the most recent addition to this group of skills. Long and Magerko define AI literacy as "[A] set of competencies that enable individuals to critically evaluate AI technologies, communicate and collaborate effectively with AI, and use AI as a tool online, at home and at work." (Long/Magerko 2020:1).
According to Markauskaite et al., “there is little doubt that AI reconfigures the distribution of intelligence, labour and power between humans and machines, and thus new kinds of capabilities are needed […].” (Marcus Kaite et al. 2022:2). These capabilities are often discussed under the term "AI literacy".
If you look at the topic of AI from the perspective of skills, there are different approaches. One question could be which human skills could become obsolete and which skills could be lost in humans because the work is done by language models. E.g, Sandrini has developed the so-called ‘translator obsolescence cycle’, which depicts the potential loss of certain skills as the degree of automation increases. Another recent study by Ayvazyan et al. examines which skills remain largely unaffected by large language models and could become increasingly important for human translators; this shift could mean higher qualifications in the profession in the long term. This echoes Olohan's statement in relation to neural machine translation: “[A]t least in the foreseeable future, it seems appropriate to think about the increasing use of technology as being frequently accompanied by an upskilling of translators, which is reflected in the need for translators to receive specific postgraduate training and education” (Olohan 2017:277–278).
From this perspective, the technologies that are currently available have potential, but they require the integration of people into quite complex work processes.
The AI Literacy Framework for Translation, Interpreting and Specialized Communication aims to reflect this upskilling approach and focuses on modern large language models as well as new technologies that could follow on from these models. The framework examines the impact of these technologies on translation, interpreting and specialized communication from a competence perspective - and also from a university teaching perspective to answer the question of what future students should learn in this field.
Overall AI literacy is divided into several dimensions: technical foundations, domain-specific performance, interaction, implementation and ethical and societal aspects, as illustrated in Figure 2.
Technical foundations
The technical dimension includes understanding how modern AI technologies and artificial neural networks work, especially the Transformer architecture with its self-attention mechanism and the use of word and sentence embeddings. Aspects such as the training methods of these networks, the training pipeline, fine-tuning and bringing the models into line with certain human values are also included. Another important aspect is the difference between natural and synthetic training data and their effects. It is crucial to understand that in modern AI technologies, the synthesis of AI model and training data forms an inseparable whole, where the weights of the model are calculated based on the training data - comparable to an omelette that cannot be broken down into its original ingredients. In addition, the technical dimension also includes the watermarking of AI-generated content, which is particularly important with regard to potential manipulation.
One might wonder why such technical foundations are necessary in the context of translation, interpreting or specialized communication and are not only relevant for computational linguists. A quote from Dorothy Kenny illustrates this point well: she emphasizes that working with "black box" technologies such as AI models without a basic understanding of how they work can lead to disempowerment of those who use these technologies. As long as we don't know what these technologies can do and what their limitations are, they work like magic - and this can prevent us from critically questioning their promises. A solid technical foundation helps to counter claims that promote new technologies as a panacea and enables us to use these technologies responsibly and critically.
Domain-specific performance
The domain-specific performance dimension is about the scope of the capabilities of these models. It concerns the level of performance for specific tasks, the identification of human added value, input-output modalities, their future potential and potential machine circularities. Determining the scope of capabilities and the task-specific performance level is not as trivial as it sounds, as the capabilities of these AI technologies remain somewhat opaque due to their general-purpose character (see figure 1 above).
An important point here is: what is the human added value? We need to ask ourselves this question in the future - not only for translation, but also for other professional fields in which these models perform impressively. For example, if a task cannot currently be automated by GPT - what happens when GPT-4 or a future version such as GPT-5 can take over this task? There are also dangers, such as those of introducing machine circularities, because these models are so versatile that one and the same model could produce a text, machine pre-process, translate and post-process that text, and finally perform a quality assessment of that text. This is theoretically possible, but from a risk diversification perspective, it is better to distribute these processes across multiple technologies and, if possible, multiple human experts. This is also where the concept of the "expert in the loop" comes into play, in which humans are involved in AI-assisted workflows in various ways remain responsible for the final quality of a product.
Psychologist Daniel Kahneman makes a general distinction between fast and slow thinking. There is one system for fast, instinctive, automatic thinking that requires little to no cognitive effort, and another for slow, rational thinking that involves more complex decisions. Andrej Karpathy and others have drawn this parallel with LLMs. They say that LLMs excel at thinking quickly and effortlessly. However, they are less capable of more elaborate, abstract and complex thinking that humans excel at. So if you ask about the overall added value of humans, this can be found above all in their ability to think in complex, abstract ways.
As Bubeck et al put it: “[GPT-4] relies on a local and greedy process of generating the next word, without any global or deep understanding of the task or the output. Thus, the model is good at producing fluent and coherent texts, but has limitations with regards to solving complex or creative problems which cannot be approached in a sequential manner.” (Bubeck et al. 2023:80).
OpenAI has a new series of models, the so-called "o1 series", in which the models are trained to go through a "chain of thought" before answering a question, in which the model breaks down a complex task into specific subtasks and then processes them one after the other. It has been shown that the "thinking skills" of this o1 series have been improved by this internal “chain of thought”. However, it is still unclear how far these models can be further developed in this respect.
Interaction
The interaction dimension covers knowledge of the available modalities of interaction, such as writing, speaking and potentially gesture control. It also includes specific pre- and post-editing, prompting, as well as the cognitive level, which concerns changes in the areas of receptive or productive skills or cognitive effects in a hybrid human-AI system. This also includes the development of adaptive expertise or a macro-strategy. The action level is also an important component of this dimension.
This dimension is particularly important from a human perspective. The question arises as to how to interact appropriately with these models in certain usage contexts and for specific tasks in which these models are intended to provide support. This requires knowledge of the modalities of interaction that are available today. Currently, we mainly write text-based prompts, but if you use OpenAI on a smartphone, for example, you can simply talk to the model. Future widespread interaction with these models may therefore no longer rely solely on written input.
Interaction may also include AI-specific pre- and post-editing beyond the field of MT. For example, one could imagine that developer documentation for a technical assembly or machine is entered into the AI and GPT then converts this documentation into user documentation. This would then be post-edited by a human expert.
There is also the cognitive level of interaction, which deals with possible changes in the areas of receptive or productive skills of AI users. The ideal notion is that of humans and machines working together and complementing each other. Ideally, our weaknesses are complemented by GPT and vice versa, but in practice this is not always the case. There could also be potential negative effects, such as stagnation in competence development or de-skilling, if too much reliance is placed on AI output. There is some evidence from machine translation research that translators who post-edit machine-translated text often still retain traces of the machine output. This issue will probably also play a role in the interaction with LLMs.
The same applies to the level of action. How is the ability to act distributed between humans and machines? There are concepts of collaborative agency that ultimately aim to expand the agency of both humans and machines so that they work together as one system.
The term "prompting" covers actions that humans use to guide LLMs to perform the intended tasks. Prompting is increasingly seen as an expert skill and is not trivial. A study by Zamfirescu-Pereira et al. showed how non-AI experts designed their prompts opportunistically and not systematically. They tended to interact with LLMs as one would interact with a human, without specifically considering that they were working with large language models. This led to overgeneralization, where solutions from one context were applied to others, often with ineffective results.
The OpenAI website has strategies and tactics for prompt engineering that show that there is more to prompting than meets the eye. Also, White et al. have developed a prompt pattern catalog that originated in software programming. Andrej Karpathy argues that the hottest new programming language is English, which means that this natural language interaction can be seen as a kind of programming where you instruct the LLM to perform certain tasks.
Implementation
The implementation dimension includes the establishment of an AI culture, the selection of an AI model, quality control, economic aspects, AI risks and legal frameworks applicable to AI. It deals with the question of how these systems can be integrated into practical workflows, which is much more difficult than simply buying a ChatGPT subscription and squeezing the model into existing workflows, without taking the concerns of end-users (translators, technical editors or other employees) into account.
The first step is to establish an AI culture within the company. In a study by Salesforce, more than half of generative AI users in international companies stated that they use AI tools without formal authorization. They reported that there are no guidelines from their employers on how to use these technologies, and many would have liked to receive corresponding training. This means that the AI culture was not yet established in these organizations - although things may have changed in the meantime. An AI culture requires a basic understanding of what tasks and problems in the organization could be solved by AI and how this can be done.
Many questions need to be answered, such as what processes need to be designed or redesigned, what the general level of automation is, where human added value is needed and which tasks could potentially be fully automated. And how does the European Union's new AI legislation fit into this context? There are ergonomic factors, such as organizational and cognitive ergonomics, that should be considered in order to introduce and implement AI adequately and in a responsible manner.
An AI provider must then be selected based on the appropriate AI model. There are thousands of models that can potentially be used: Closed source, open source, large or small models, and those that excel in different languages.
Translation studies and the translation profession are particularly well-versed in AI implementation, as they have been dealing with neural machine translation (NMT) at least since the invention of the transformer in 2017. They know how to design or not design processes when integrating NMT and are familiar with the various risks associated with NMT. Many of these insights from translation studies could be integrated into other workflows where language models are used.
Ethical and societal aspects
Ethical and social aspects constitute the final dimension of the model and go beyond the narrow focus on specific professional fields. This dimension deals with the question of what AI skills we need beyond our professional role as citizens in an AI-saturated society. For example, there is the question of whether AI could bring about social empowerment or disempowerment. Translators have already had unfortunate experiences of social disempowerment through NMT and other technologies - be it economic, loss of social status or loss of cultural capital. There is also a risk that these models will produce toxic results, as they are very powerful and can answer a variety of questions that a human would not be expected to answer. Safety barriers must therefore be put in place to avoid these toxic results. Another risk is manipulation. AI models are pretty good at mimicking voices. Robo-calls or emails created with this technology could trick people into accepting assignments that offer poor compensation and short deadlines, or they could be used to manipulate translators or interpreters. There is also a risk of epistemic violence or bias. AI models tend to misrepresent reality and reproduce social biases, such as gender bias. There is also a tangible and intangible substrate on which these AI technologies are based. They did not develop in a vacuum, and there are environmental costs. A significant amount of intellectual work has gone into developing the algorithms and compiling the training data. Again, these aspects have already been reflected by translation studies, which could also make important contributions to technology impact assessments with regard to the actual consequences of the implementation of these technologies at a societal level.
Planned didactic operationalisation of the framework
The next step involves the didactic operationalization of the framework in the spirit of the learning resources created by the DataLitMT project. One potential vehicle for this operationalisation is the European Union Erasmus+ consortium "LT Leader: Language and Translation: Literacy in Digital Environments and Resources". The aim of the consortium is to map the technological landscape in the translation sector and to create learning resources for the development of digital skills in relation to these technologies. The focus of these digital skills is, of course, on AI skills. This is because the technological landscape is becoming more and more dominated by large language models.