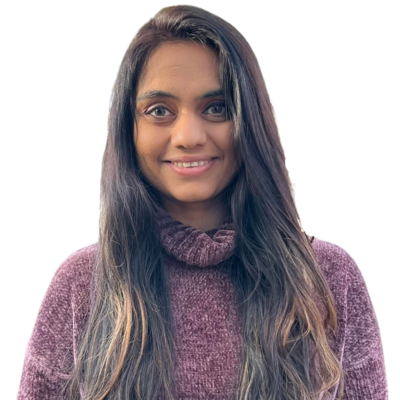
Human augmentation in Technical Communication in the world of AI
A summary of the IUNTC Talk on February 29, 2024, by Nupoor Ranade
Abstract of the talk: In the talk, I plan to discuss practical ways for technical and professional communicators to engage in ethical AI development using familiar approaches such as User experience design, Human-in-the-loop using AI augmentation and Communicating for AI transparency. The humanities and AI are two fields that have traditionally been seen as separate and distinct. However, in recent years, there has been a growing interest in the intersection of the two. This is due in part to the increasing sophistication of AI systems, which are now capable of performing tasks (such as writing and editing, especially for creative purposes) that were once thought to be the exclusive domain of humans. Another aspect is the rising ethical concern about AI technology and its impact on society. Humanities scholars like technical communicators can get involved in the research on AI ethics in different ways. Some of those are as follows: 1) they can collaborate with technical experts to help breakdown complex concepts for easier interpretability, 2) they can use their community engagement skills for user and public advocacy, and 3) they can use critical methods to evaluate the ethical impact of AI to check for biases. I will demonstrate these ideas by describing my work on five different projects to highlight the scholarship in rhetoric, technical communication, and other humanities fields in general, and to invite relevant discussions.
Dr. Nupoor Ranade is an Assistant Professor of English at George Mason University. Her research addresses knowledge gaps in the fields of technical communication practice and pedagogy, and focuses on professional writing and editing, inclusive design, and ethics of AI. Through her teaching, Nupoor tries to build a bridge between academia and industry and help students develop professional networks while they also contribute to the community that they are part of. Her research has won multiple awards and is published in journals including but not limited to Technical Communication, Communication Design Quarterly, Kairos, and IEEE Transactions on Professional Communication.
March 2024 - written by Yvonne Cleary & Daniela Straub
Read full article
The Ambivalence of AI
Human augmentation explores collaboration with technology to enhance physical or cognitive abilities. However, the ambivalence of AI for humans becomes evident when we consider its differing perceptions in Sciences and Humanities. In scientific circles, AI is viewed as groundbreaking and revolutionary, largely because of opportunities and advances in research. This positive, transhumanist perspective emphasizes that humans should use technology to develop enhanced abilities beyond their natural limits. Consequently, the goal in technology development is to create "superpowers" that can significantly expand individual potential.
In the Humanities, there is concern about the impact of AI on traditional professional fields. The Humanities engagement with AI reflects on the relationship between humans and technology and how this collaboration can be improved. Specialists in writing and editing fear that automation threatens their prospects in content generation and social media communication. The younger generation in English studies is worried about their professional future in light of these developments.
This disparity of perspectives underscores the need for a nuanced examination of the impacts of AI. It is essential to address concerns in various fields while simultaneously recognizing and promoting the positive potential of AI.
Risks of Using AI
A crucial risk is the consequence of AI deployment, and the apparent lack of accountability. The impacts on societies worldwide could be significant when no accountability is established. Questions about transparency, the boundary between truth and falsification, ethical responsibility, and accountability in collaboration are central. How can we ensure that information is accurately conveyed, and the population learns to discern right from wrong, especially when users lack experience or context?
Governments and regulatory bodies have developed different approaches: the European AI Act emphasizes a risk-preventive approach, requiring developers and companies to disclose information about the risks of AI products. The UK's AI Action Plan emphasizes clear communication of the design of AI technologies to promote innovation. The US Executive Order focuses on data privacy, civil rights, consumer protection, innovation, and taking responsibility to ensure the safety and trustworthiness of AI technologies.
Ensuring safety and trustworthiness raises the question of how to promote understanding both of what the technology is capable of and also how it works. Trust requires communication, and technical communication plays a crucial role in the development and communication process of AI. If technical communication does not actively intervene in the development and communication of AI, numerous opportunities will be missed to make valuable contributions to the development of AI. Therefore, increased involvement of technical communication in the machine learning development cycle and reconsidering ways in which this can happen are urgently needed.
Human augmentation of technical communication for AI
Technical communication involves the ability to provide clear, consistent and factual information, especially when dealing with complex concepts. Technical communicators translate complex ideas into simple language. Customizing content for specific audiences ensures that the content is understandable for different users. The documentation development lifecycle plays a central role here, starting with planning and analyzing the target audience, followed by developing content using various tools and technologies. This is where the diverse contributions of technical communication in the development and use of AI and machine learning come in.
Technical Communication for AI Documentation
An important area where technical communication can contribute is in the documentation of AI, particularly addressing the complexity of ‘black box’ ideas. Increasing access and transparency of these complex ideas is important, both for users and regulatory authorities. For example, the introduction of bias in AI generated content is a concern. The discussion of how to avoid bias began in 2018 with the idea of documenting the technology development process in detail. This essentially means creating developer documentation for machine learning or AI technologies. All aspects should be captured in model cards, including training data, their composition, excluded elements, and the relevant population. The aim is to comprehensively document the entire development process of the machine learning model.
However, the reality of documentation reveals a discrepancy. Despite the rapid advancement of technology, there is a scarcity of scientific literature on AI documentation. The research literature broadly falls into three main areas: user requirements, standard development, and evaluation. Technical communication has long been engaged with these topics. However, there is often a lack of awareness in the developer and research community that this technical communication research already exists. Therefore, it is now time for technical communicators to actively engage in these discussions—not only regarding documentation as a byproduct but also concerning the development of AI technologies themselves.
Technical Communication for Prompt Optimization
The second area where technical communication can actively contribute is in rhetorical prompt engineering. This involves determining how to instruct a specific generative AI tool with a prompt to achieve the best results. Although there has been research on standards in prompt formulation for some time, there is no established formula that guarantees effective output, and this is because to-date, those addressing these issues do not come from the field of technical communication or technical writing.
The rhetorical situation, a theory from the 1960s by Bitser et al., states that the more specific or accurate one understands a communication situation, the better. Therefore, a comprehensive understanding of the current situation or problem is crucial to finding an appropriate response. This insight can be directly applied to create a formula for prompt engineering.
In an AI request, one submits the input text, the prompt, and there are various steps before seeing the output text. Due to the way machines learn, contextual embedding is crucial. Unlike human learning, where stories about situations, events, and people play a role, the machine learns by looking at word sequences or sentences that are close to each other. During the initial training of a Large Language Model (LLM) (e.g. for ChatGPT), the machine classifies words by collecting and categorizing publicly available internet content. When a request is made, the machine tries to determine which group the content falls into and then extracts relevant words and sentences. The problem with such classification systems is that there is no coherent story associated with the content. This is where human augmentation comes into play to effectively tell the machine, "Wait, let me work with you so that you give me the most accurate output."
When generating prompts, it is important to consider how the machine understands prompts. The more complete the context of the prompt, the better. Therefore, it should include rhetorical components such as: purpose, need, topic, author, and audience. Here, technical writers can leverage their knowledge of rhetorical situations to tailor information delivery for prompt engineering and develop prompt formulas for various conditions and cases.
The third area where technical communication can make a significant contribution is in the development of content itself.
The programmatic approach in teaching technical communication deals with curricular topics such as grammar, punctuation, and word selection for clarity and conciseness – areas that AI can handle well. In contrast, the rhetorical approach not only considers explicit words but also implicit contexts. For example, instead of using the term "race," the word choice "ethnicity" should be preferred, and in a software context, although it is commonly used, the word "kill" should be avoided as it evokes associations with warfare.
There is a need for more control over the process of contextual embedding of words. Technical communication can contribute by attempting to clean up data – a supervised machine learning process. Although developers can recognize problematic word pairings, teams often lack professionals who are knowledgeable about content design and can contribute to the model development process. Ethical editing and framing can be used for data preprocessing, fine-tuning, and content evaluation after the model's release. In this regard, technical communication plays a key role in ensuring the application of ethical principles and the qualitative improvement of the model.
Further Areas of Activity for Technical Communication
Technical communication can leverage implicit contributions, i.e., non-visible interactions of users, to enhance its contributions. In the software and documentation industry, technical communication uses infrastructures like GitHub to gain insights into the backend of documentation platforms and understand user issues. However, similar processes are lacking for AI tools, to understand how users use generated content and to what extent it is successful. Technical communication can contribute because we have mechanisms to understand user needs, improve products, and communicate effectively with users.
Another crucial aspect is equality and social justice. Technical communication traditionally advocates for user concerns and understands their needs and product usage. Technical communicators can contribute to creating diverse testing environments to ensure that machine learning models receive data from various relevant user groups. This contributes to more equitable and socially just technologies.